[ad_1]
In psychiatry, sex matters. In schizophrenia, women are diagnosed later than men and tend to receive less effective types of medication (Sommer et al., 2020). Furthermore, particular medication may have differential side effects between sexes and affect women more negatively (Hoekstra et al., 2021). In depression, prevalence is much higher in women than men and women tend to present with anxiety and somatic comorbidities (Eid et al., 2019). Yet, overall, men are more likely to die by suicide (Miranda-Mendizabal et al., 2019). Understanding the neurobiology of sex differences in psychiatry is vital to improve diagnostic accuracy and treatment effectiveness.
This study presents important new research into the differences in brain connectivity between males and females. This study is significant in the context of mental health as it sheds light on the fundamental ways in which brain network patterns vary across sexes, by exploring the relationship between brain networks and behavioural differences in males and females. While there have been numerous studies in this area, this paper notes that reliable sex/gender differences are few, and many of the reproducible findings are primarily driven by differences in brain size.
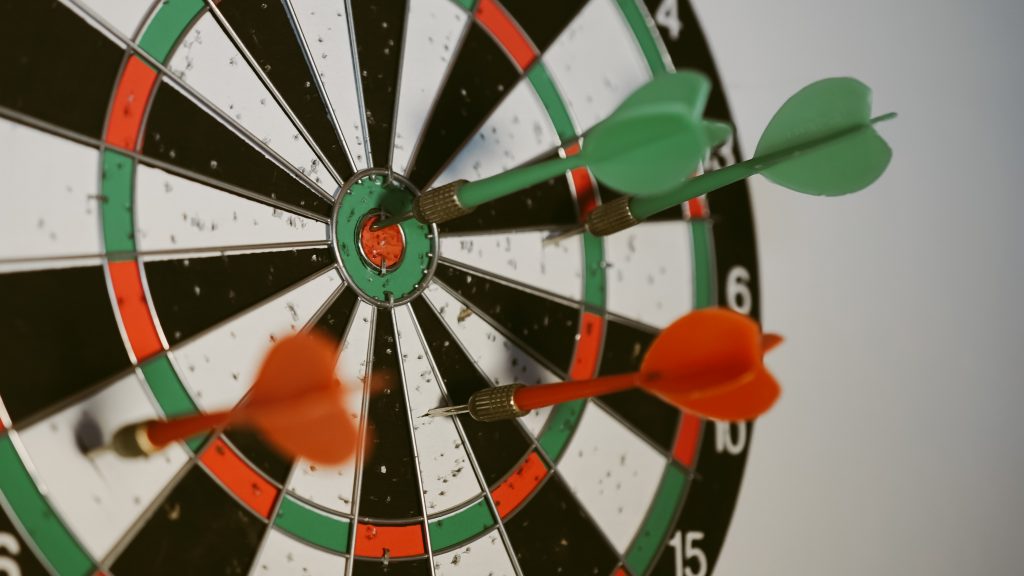
In psychiatry, sex matters. Understanding the neurobiology of sex differences in psychiatry is vital to improve diagnostic accuracy and treatment effectiveness.
Methods
This study focused on examining how individual differences in functional brain connectivity can predict the presence of psychiatric illness and the variability in associated behaviours. Specifically, they investigated the differences in functional network connectivity and their implications across sexes.
Predictive modelling approaches (linear ridge regression models) were used to assess whether shared or unique functional connectivity correlates underpin the expression of psychiatric illness–linked behaviours in males and females. This investigation was conducted using data from the Adolescent Brain Cognitive Development Study, which included a sample of 5,260 children (2,571 females) aged 9 and 10. For the assessment of behavioural data, the Child Behavior Checklist was used. It comprises eight empirically-based syndrome scales: Anxious/Depressed, Withdrawn/Depressed, Somatic Complaints, Social Problems, Thought Problems, Attention Problems Rule-Breaking Behavior, and Aggressive Behavior. These scores can then be further summarised into Internalizing, Externalizing, and Total Problems. The Internalizing domain summarises Anxious/Depressed, Withdrawn/Depressed, and Somatic Complaints. The Externalizing domains summarises Rule-Breaking and Aggressive Behaviours. Finally, the Total Problems score is based on responses to all eight of the syndrome scales.
Results
Overlapping Behaviours in Males and Females
Both sexes exhibited largely overlapping distributions of behavioural scores. However, significant sex differences were noted in several syndrome scales (e.g., withdrawn/depressed, externalising, aggressive, thought problems) and DSM-5 oriented scales (e.g., affective, oppositional defiant disorder). Males generally reported higher scores in most behaviours, with the exception of somatic complaints and problems that were higher in females.
Brain-Based Predictive Models
Linear ridge regression models trained on functional connectivity data successfully predicted various psychiatric illness-linked behaviours in both males and females. The models were more successful in predicting externalising behaviours compared to internalising ones, and this predictability varied across sexes. The use of brain-based information allowed the researchers to develop tools that were capable of detecting externalising behaviour (rule-breaking/aggressive behaviour). The accuracy of the tools was good for both sexes but higher in males.
Functional Correlates Across Behaviours and Sexes
Shared neurobiological features underlie the expression of behaviours in both sexes, despite behavioural differences. Features involved in predicting anxious/depressed behaviours and somatic complaints showed unique correlations distinct from other predictions. While males and females might present different behaviour, the neurobiology that guides that behaviour is the same across both sexes.
Behavioural scores within each sex showed strong correlations within domains (internalising and externalising), but correlations across different behavioural domains were weaker. Essentially, this shows that the domains that have been constructed reveal some internal consistency. Furthermore, the sex differences that were apparent in the behavioural data were associated with illness onset risk. This mean that these sex differences may appear before adolescence.
The authors assessed whether the brain-based models they developed were accurate when applied to a population that is different from the one that they were built on. Interestingly, brain-based models developed in males to predict one behaviour, predicted the other behaviour when applied to females. The converse effect was observed when brain-based models developed in females were applied to males. This further reinforces the point that different behaviours across sexes may be based on the same neurobiology.
Finally, functional connectivity within and between various heteromodal networks (e.g., temporal parietal, default, control, limbic, attention) were associated with specific behaviours like withdrawn/depressed and rule-breaking. These associations are largely shared across sexes.
Importantly, none of these findings could be explained by motion in the scanner, which further strengthens the arguments of the authors.
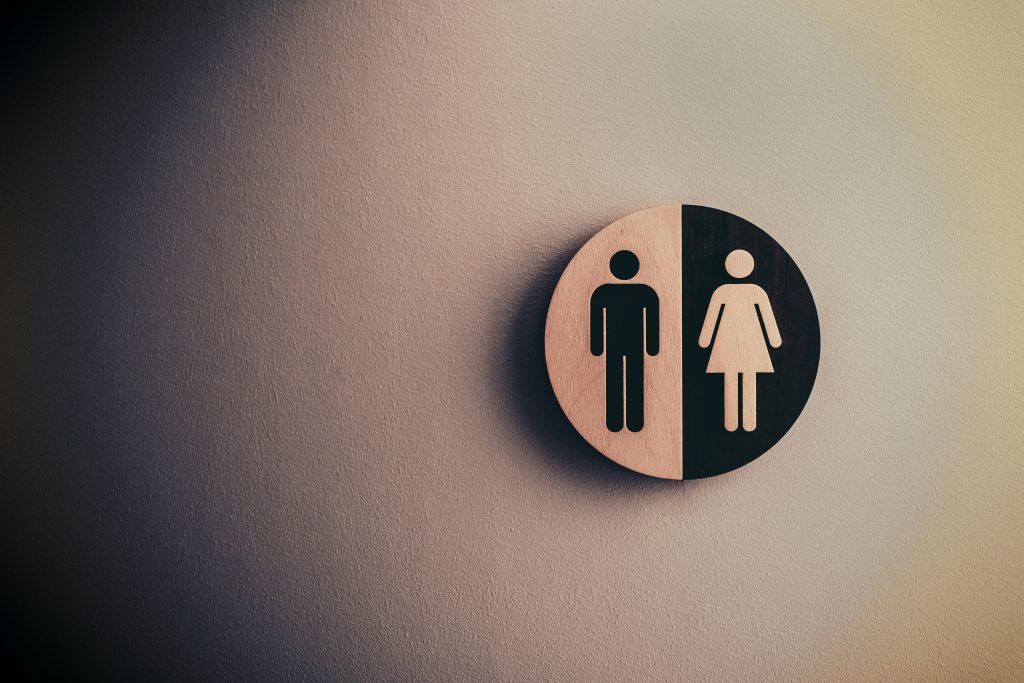
While males and females might present different behaviour, the neurobiology that guides that behaviour is the same across both sexes.
Conclusions
The authors concluded by providing critical insights into the neurobiology of psychiatric illnesses, highlighting that functional connectivity can predict certain behaviours across both sexes, but with notable distinctions. They emphasise that while externalising behaviours are predictable in both males and females, internalising behaviours are more reliably predicted in females. This finding highlights a potential sex-based divergence in the neurobiological underpinnings of psychiatric disorders.
The paper also discusses the challenges in isolating disorder-specific biomarkers due to the overlapping nature of psychiatric conditions and the high comorbidity rates. Importantly, it suggests that while psychiatric illnesses manifest differently in males and females, there are shared neurobiological features across sexes that contribute to these illnesses. This insight could be pivotal in developing more effective, personalised treatments and diagnostic tools that transcend traditional categorical approaches and consider the nuances of sex differences in psychiatric presentations.
The authors rightly acknowledge the study’s limitations, such as the focus on a child cohort and the exclusion of gender identity and socio-economic factors, hinting at the complexity and evolving nature of psychiatric research.
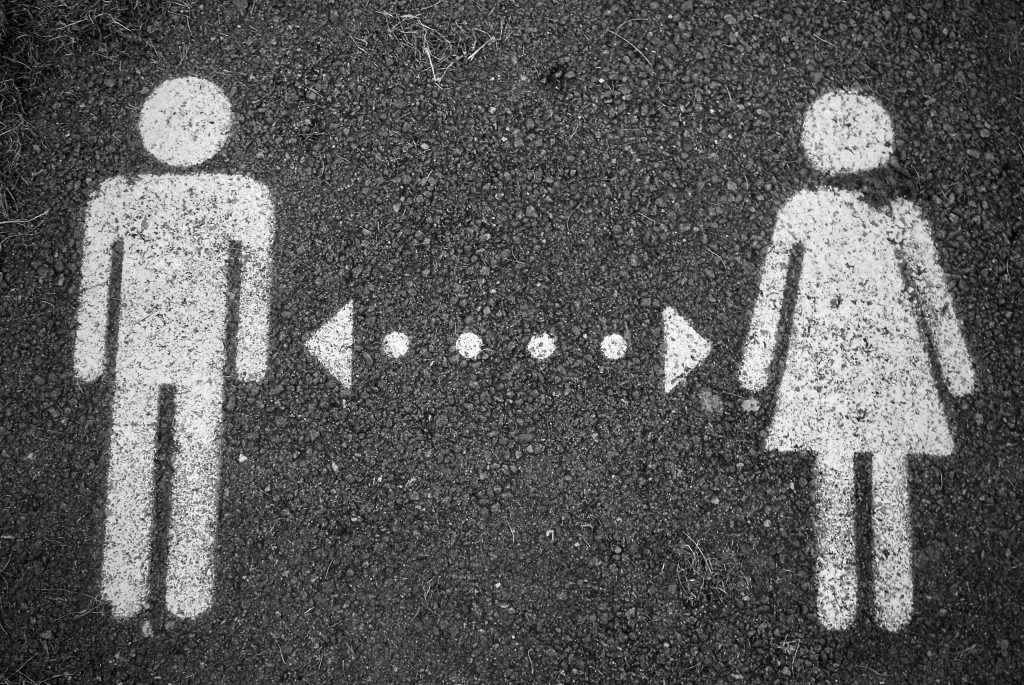
While psychiatric illnesses manifest differently across sexes, some neurobiological features are shared. This insight may lead to more effective, personalised treatments and diagnostic tools that transcend traditional approaches and consider the nuances of sex differences in psychiatric presentations.
Strengths and limitations
Strengths
Large Sample Size
A significant strength of the study is its large sample size, comprising 5,260 children. This extensive dataset provides a robust foundation for the analysis, enhancing the reliability and generalisability of the study’s findings. The substantial number of participants allows for a more nuanced understanding of the neurobiological correlates of psychiatric illness-relevant behaviours across different sexes.
Strong Rationale and Important Topic
The study is underpinned by a strong rationale, addressing the critical need to understand the neurobiological underpinnings of psychiatric illnesses and behaviours, particularly in the context of sex differences. This is an important and timely topic, given the growing awareness of sex-specific differences in psychiatric disorders. The research contributes valuable insights into the development of personalised preventive interventions, diagnostic procedures, and therapeutic modalities, which is crucial for advancing mental health care.
Limitations
Lack of Detailed Information on Model Development
The study does not provide detailed information regarding the development of its predictive models, particularly in aspects such as hyperparameter optimisation and preprocessing parameters. This lack of detail suggests that there may not have been a repeated nested cross-validation framework, which is crucial for validating the predictive performance of the models and ensuring their robustness (Varma et al., 2006).
Motion Parameters Not Correlated with Prediction Metrics
A key limitation of the study is the apparent absence of correlation between motion parameters and prediction metrics. This oversight could mean that the potential influence of motion artifacts on the predictive accuracy of the models has not been adequately addressed (especially considering that the population at hand are ages 9 and 10). Motion artifacts in neuroimaging data can significantly impact results, and their potential contribution to prediction outcomes should be carefully assessed (Scheinost et al., 2019).
Absence of Robust Site Correction
The study does not appear to implement robust site correction methods. In multi-site neuroimaging studies, such as this one, varying data acquisition protocols across different sites can introduce systematic biases. The absence of robust site correction techniques could lead to confounding effects, potentially compromising the validity of the findings (Scheinost et al., 2019).
In summary, while the study boasts a large sample size and addresses an important topic with a strong rationale, it is limited by gaps in methodological details, particularly in model development and correction for potential confounding factors like motion artifacts and site variations. These limitations could impact the validity and applicability of its findings in clinical settings.
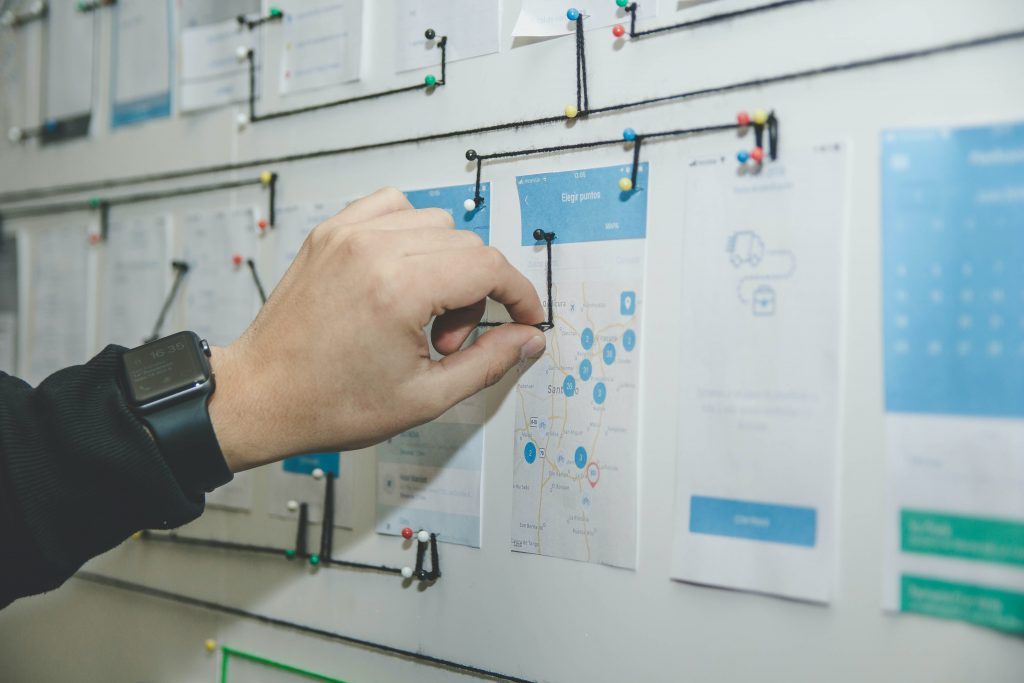
This study boasts a large sample size and addresses an important topic with a strong rationale, but it is limited by gaps in methodological details, impacting the validity and applicability of its findings in clinical settings.
Implications for practice
This study’s findings highlight the predictive capacity of functional connectivity profiles for externalising behaviours in both sexes and internalising behaviours predominantly in females. It shows significant promise for refining diagnostic and therapeutic approaches in psychiatry. Identifying sex-specific approaches could facilitate more accurate identification of at-risk individuals, particularly children, and enable early intervention strategies tailored to their specific neurobiological profiles. Additionally, the study underscores the necessity for clinicians to be cognizant of sex differences in psychiatric presentations. Understanding that certain behaviours may manifest differently across sexes due to underlying neurobiological factors can enhance the accuracy of diagnoses and the effectiveness of treatments.
Furthermore, the evidence presented opens up several new research avenues.
- There’s a need for longitudinal studies to track the progression of psychiatric disorders across different developmental stages, particularly focusing on how sex-specific neurobiological correlates evolve over time. Such longitudinal data can shed light on critical periods for intervention and the long-term efficacy of treatments tailored to neurobiological profiles.
- Research should delve deeper into understanding the mechanisms underlying the observed sex differences in brain connectivity and psychiatric presentations. Studies could explore the interplay between genetics, hormones, and environmental factors in shaping these differences.
- The study highlights the potential of machine learning and AI in psychiatry, specifically in predictive modelling based on neuroimaging data. Future research should focus on refining these models, exploring their utility in diverse populations, and extending their application to a broader range of psychiatric conditions.
In conclusion, the implications of this study hold significant promise for necessitating changes in clinical practice and future research directions. Embracing these changes could significantly enhance our understanding and management of psychiatric disorders, leading to more personalised and effective mental healthcare. Currently, most research of mental health problems tends to view sex as a confounder that needs to be regressed out of the signal of choice in order to identify “pure” disease-related signals. However, more and more research in the last few years shows us that sex is intrinsically related to differential disease progression patterns and, as this study shows, related to unique neurobiological pathways of disease.
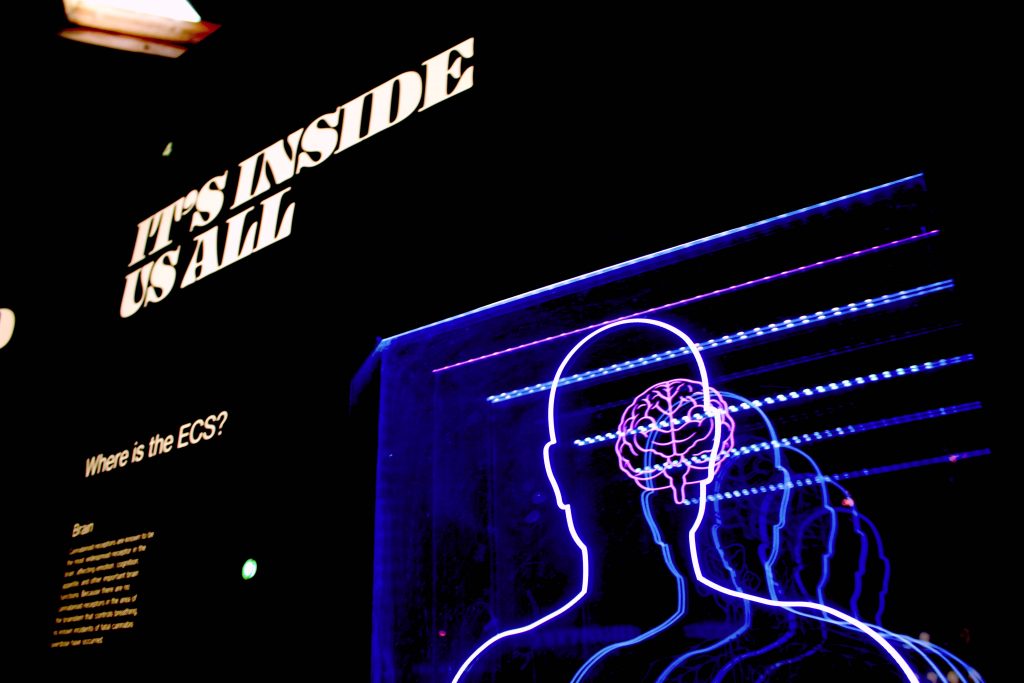
Understanding that certain behaviours may manifest differently across sexes due to underlying neurobiological factors can enhance the accuracy of diagnoses and the effectiveness of treatments.
Statement of interests
None
Links
Primary paper
Dhamala E, Rong Ooi LQ, Chen J, Ricard JA, Berkeley E, Chopra S, Qu Y, Zhang XH, Lawhead C, Yeo BTT, Holmes AJ. Brain-Based Predictions of Psychiatric Illness-Linked Behaviors Across the Sexes. Biol Psychiatry. 2023 Sep 15;94(6):479-491. doi: 10.1016/j.biopsych.2023.03.025
Other references
Eid, R. S., Gobinath, A. R., & Galea, L. A. M. (2019). Sex differences in depression: Insights from clinical and preclinical studies. Progress in neurobiology, 176, 86–102. https://doi.org/10.1016/j.pneurobio.2019.01.006
Hoekstra, S., Bartz-Johannessen, C., Sinkeviciute, I., Reitan, S. K., Kroken, R. A., Løberg, E. M., Larsen, T. K., Rettenbacher, M., Johnsen, E., & Sommer, I. E. (2021). Sex differences in antipsychotic efficacy and side effects in schizophrenia spectrum disorder: results from the BeSt InTro study. NPJ schizophrenia, 7(1), 39. https://doi.org/10.1038/s41537-021-00170-3
Miranda-Mendizabal, A., Castellví, P., Parés-Badell, O., Alayo, I., Almenara, J., Alonso, I., Blasco, M. J., Cebrià, A., Gabilondo, A., Gili, M., Lagares, C., Piqueras, J. A., Rodríguez-Jiménez, T., Rodríguez-Marín, J., Roca, M., Soto-Sanz, V., Vilagut, G., & Alonso, J. (2019). Gender differences in suicidal behavior in adolescents and young adults: systematic review and meta-analysis of longitudinal studies. International journal of public health, 64(2), 265–283. https://doi.org/10.1007/s00038-018-1196-1
Scheinost, D., Noble, S., Horien, C., Greene, A. S., Lake, E. M., Salehi, M., Gao, S., Shen, X., O’Connor, D., Barron, D. S., Yip, S. W., Rosenberg, M. D., & Constable, R. T. (2019). Ten simple rules for predictive modeling of individual differences in neuroimaging. NeuroImage, 193, 35–45. https://doi.org/10.1016/j.neuroimage.2019.02.057
Sommer, I. E., Tiihonen, J., van Mourik, A., Tanskanen, A., & Taipale, H. (2020). The clinical course of schizophrenia in women and men-a nation-wide cohort study. NPJ schizophrenia, 6(1), 12. https://doi.org/10.1038/s41537-020-0102-z
Varma, S., & Simon, R. (2006). Bias in error estimation when using cross-validation for model selection. BMC bioinformatics, 7, 91. https://doi.org/10.1186/1471-2105-7-91
Photo credits
[ad_2]
Source link